The Challenges of Implementing Data Science Methods to the Cybersecurity Practices of National Power Grids.
My dissertation research project marking the completion of my MSc in Data Science.
I spent 3 months deliberating whether to study blockchain technologies being applied to political voting systems (liquid democracy) or the challenges of applying Cyber Security Data Science (CSDS) in a real-world context. I opted for the latter and spent the next 9 months pre reading around the applications of CSDS within national power grids.
Whilst working a full-time job as a Forecasting Data Analyst, I mainly worked on the dissertation in my spare time at the weekend and after post work climbing sessions. Fuelled by Club Mate, The Hop Cafe CBD Coffee's, and an insatiable curiosity for this field - I thoroughly enjoyed writing this dissertation.
Why Power Grids?
I watched a really cool vice documentary on the future of warfare with cyber attacks targeting the critical infrastructure of countries (it has since been removed from youtube).
Acknowledgements
I am truly grateful for the opportunity to be able to conduct a research project like this, combining my passion for data science, cyber security, and human innovation. I would like to express my appreciation to all those who made it possible.
Thank you to the academic staff at the University of Sheffield for fostering my passion for Data Science and pushing my intellectual grasp beyond what I thought possible, Dr. Lyubo Mishkov, Jonathan Jeffery, Dr. Olga Cam, Dr. Morgan Harvey, Dr. Suvodeep Mazumdar, and Dr. Peter Stordy.
A special thanks to Dr. Jo Bates who provided space for creativity and valuable support throughout this research project. Inspiring me to take my next steps after academia.
This research project would not have been possible without my loving family, Jenny, Walter, Johnathan, Clare, Walter Sr., Marie, and Stephen. Thank you to all of my supportive, intelligent, and caring friends who inspire me every day, Evan, Conor, Jack, Meg, Jess W., Mica, Ashleigh, Jess M., India, Lucy, and Georgia. Also, my dear therapist Maggie.
Thank you to my family and friends for always believing in me, even during times when I was unable to believe in myself. I cherish you all and would not be where I am today without you.
Abstract
Background
With the rapid expansion of internet of things (IoT) technologies and data science methods, power grids have needed to adapt their cybersecurity measures in this new era of datafication - to defend critical infrastructure. The risk to critical infrastructure is increasingly a digital risk. Specifically, foundational infrastructure, which is integral to the widespread functioning of contemporary Western civilisation, is ever more dependent on data-driven and algorithmic systems deployed by data scientists. This dynamic raises questions, and this research aims to provide an investigation into such questions.
Aim
To investigate the challenges, benefits, and consequences of the application of data science methods to cybersecurity practices of national power grids.
Methods
A policy review was conducted on 8 documents, accessed from the Overton database. Two semi structured interviews with cybersecurity professionals were utilised. NVivo software was deployed to perform thematic analysis to reveal themes discovered from the 8 policy documents and 2 interview transcripts.
Results
Thematic analysis unveiled 4 themes: Critical Infrastructures, Cybersecurity Organisations, Cybersecurity Technique(s) and, Data Science Methods. Within the data science methods theme were 5 more sub-themes that revealed 5 challenges in the adoption of data science methods within the context of a power grids’ cybersecurity (AI threats, Human Judgement, Lack of Policy, Sharing of Data, Time Sensitivity). AI threats was observed across every document analysed. Human judgement occurred most in the interview transcripts (N=17). Time sensitivity was the most common sub-theme found in the analysis, occurring in 5 out of 8 policy documents and in both interview transcripts (N=48).
Conclusion
The themes discovered were critically analysed with existing literature, 5 significant challenges were found regarding the implementation of data science methods to cybersecurity practices of national power grids. Resulting in 2 principled recommendations for policies makers to consider and 3 areas identified to conduct further research in.
Introduction
We live in a digital age. One can evidence this by looking to the rapid, technological evolution happening within two distinct but intimately related disciplines, artificial intelligence (AI) and machine leaning (ML). It is rapid because the curve of progress has been calculated as exceeding the rate suggested by Moore's Law (Perrault, 2019).
To quantify this, with research that is now nine years old, and therefore underestimates the numbers, there is evidence suggesting that 2.5 quintillion bytes of data are produced by the human species every single day, which suggests that almost all aspects of daily life is becoming digitised(Lu, Zhu, Liu, Liu, & Shao, 2014).
Increasingly, this digitisation drives decision making, guides social interaction and revolutionises societal infrastructure(Kitchin, 2021). The symbioses of data, society, and infrastructure has led cybersecurity to be a crucial discipline for every aspect of modern-day life(Soni, Kaur, Gupta, Sharma, & Gupta, 2023). In developed countries, the infrastructure of power grids that supports this digital era of computers, databases, IoT, servers, and networks is now a potential attack vector(Ou, Zhen, Li, Zhang, & Zeng, 2012). The practice of data science (DS), which helped gain insight from this vast amount of data generation, is today being deployed specifically to protect digital infrastructure that broader society is now inextricably linked with.
The technology producing such vast amounts of data is underpinned by wireless sensor networks (WSN), which allow us to measure, infer, and understand environmental indicators with the intercommunication of WSN’s created the IoT(Gubbi, Buyya, Marusic, & Palaniswami, 2013). One can define the IoT as the interconnection of the physical world of things with the virtual world of cyber space(Mazhelis, Luoma, & Warma, 2012). Included in that physical world of things is national power grids, now being integrated to form smart or intelligent power grids(Han, Zhang, Zhang, & Gu, 2010). While there are many benefits for countries integrating these smart grid technologies, such as reduced damage of natural disasters to transmission lines, reliability of power, and reduced economic loss(Ou, Zhen, Li, Zhang, & Zeng, 2012), these benefits also come with the cost of their critical infrastructure being exposed within cyber space.
Considering the expansion of IoT to include national power grids, and their integral nature to a nation’s infrastructure, cyber-attacks are now deemed an extreme physical threat in the context of IoT(Ahmad & Zhang, 2021). What was once a hypothetical threat is today an immediate one. That is, the danger that an agent attacking such systems could disrupt a country’s ability to operate and supply electricity to its citizens is relevant, real, and calls for proactive response (Komninos, Philippou, & Pitsillides, 2014). For instance, recent attacks in Pakistan and India caused temporary large-scale blackouts(Krause, Ernst, Klaer, Hacker, & Henze, 2021). As national power grids become more reliant on IoT they become more vulnerable to computerised attacks. Therefore, it is vital we enhance our cybersecurity solutions to overcome these threats and protect IoT devices including smart power grid networks(Bedi, Kumar Venayagamoorthy, Singh, Brooks, & Wang, 2018).
The threats considered, what is needed is a clear, efficient, and proactive approach to address these vulnerabilities. What might be helpful are the plethora of cyber vulnerability data, which can provide important insights(Tang, Alazab, IEEE, & Luo, 2019). Given the multivariate nature of complex cyber-attacks, ignoring this data could lead to severe underestimation of cybersecurity risks(Peng, Xu, Xu, & Hu, 2018). To handle these threats data science and cybersecurity are now collaborating to create novel solutions for this emerging threat.
Research Question, Aims and Objectives
Research Question
As power grids have been defined as critical infrastructures to countries, their security is paramount(European Union, 2023). Focusing on their cybersecurity in the context of ongoing digitization, introducing data science techniques to these cyber security practices leads to the following research question:
“What are the challenges posed with the adoption of data science within cybersecurity practices of national power grids?”
Krause, Ernst, Klaer, Hacker, & Henze(2021)have suggested that as networks within power grids expand this can lead to several vectors that attackers can target. Although Krause et al.(2021)provide suggested solutions for device and application security, network security, physical security and policies, procedures & awareness, there is little research into the challenges faced when these cyber security data science practices are implemented.
Research Aim
As the research question suggests, there is a need to investigate the challenges associated with the application of cybersecurity data science methods to national power grids. Doing this will enrich the research literature within this area. Alongside the research question is a research aim, which is:
“To investigate the challenges, benefits and consequences of the application of data science methods to cybersecurity practices of national power grids.”
Research Objectives
This research question and aim will be achieved with some logical research objectives which are:
1. To identify the key issues relating to cybersecurity of national power grids and how data science can help with combatting these issues through a review of current literature.
2. To investigate themes within current policies of cyber security practices of US national power grids through a policy analysis, to identify any present challenges.
3. To further contribute to the discussion of data science practices being applied to cyber security through interviews with professionals within the cybersecurity industry.
4. Provide a framework or make principled suggestions which policy makers could follow to ensure proper implementation of CSDS and address any identified challenges.
Definitions
Data Science
On the whole data science (DS) aims to improve decision making through the analysis of data with a broad range of computational tools(Kelleher & Tierney, 2018). Driving most decision making in modern day society, encompassing set principles, algorithms, and processes for uncovering insight. Formally recognised as an emerging discipline in the early 2000’s by academic journals(University of Wisconsin Data Science Team, 2022), the rapid growth of the field has been fuelled by insurmountable metadata produced through social media platforms and communication services(van Dijck, 2014).
Cybersecurity
Cybersecurity’s (CS) definition is “highly variable, context-bound, often subjective, and, at times, uninformative”(Craigen, Diakun-Thibault, & Purse, 2014). This study will use the definition proposed by Lewis(2006)which defines cybersecurity as the safeguarding of computer networks and the information they contain, from penetration and other malicious damage or disruption. The constant innovation of this field is imperative to defending computer networks critical to countries infrastructures from a plethora of attacks(CSIS, 2023). A cyber-attack, according to Hathaway, et al.(2012), would be anything that threatens the functions of a computer network for a political or national security purpose.
Within cyber security there are many defence mechanisms for protecting a computer network system. However, this study will focus particularly on intrusion detection systems (IDS) as defined by Anwar, et al.(2017). This team of researchers posited that to define IDS, one is talking about hardware or software systems that automatically identify and respond to attacks on computer systems. Traditional methods of CS include Firewalls, which secure the front access points of a network connected node from a number of threats and attacks. Access control is deployed for authentication purposes through two or three factor authentication of the user. Cryptography as pioneered by Turing(1942), allows for communication of data to be secure and only decrypted by known recipients.
National Power Grids and Smart Grids
Here, the word “grid” is defined as an electricity system that may perform some or all of the following operations: electricity generation, electricity transmission, electricity distribution and electricity control(Fang, Misra, Xue, & Yang, 2011). Traditionally, these systems are one-way networks where generators produce electricity. This energy travels across high-voltage transmission lines to arrive at a distribution network, which delivers electricity to consumers(Nardelli, et al., 2014).
Smart grids are defined as the intersection between IoT and the traditional aforementioned power grid(Han, Zhang, Zhang, & Gu, 2010). Because of differences in their functionality, the architecture which smart grids offer not only is distributed with more physical layers within the system, but it is also managed by adaptive controls and smart algorithms utilising ICT(Palensky & Kupzog, 2013).
National power grids and smart grids are both considered to be crucial for society to function hence being recognised as critical infrastructure, with other examples of critical infrastructures being health care systems, financial markets, railway systems etc.(Venkatachary, Prasad, & Samikannu, 2017).
Internet of Things (IoT)
IoT can be defined as the interconnection of the physical world of things with the virtual world of cyber space(Mazhelis, Luoma, & Warma, 2012). The IoT network consists of millions of private, public, academic, business, and government networks, on a local and global scale all of which are linked by a broad array of electronic, wireless, and optical networking technologies(Madakam, Ramaswamy, & Tripathi, 2015)such as the WSN’s mentioned in the introduction.
Cybersecurity Data Science (CSDS)
Cybersecurity data science (CSDS) is defined by Sarker, et al.(2020)as data focused security. It applies machine learning methods to quantify cyber risks, and ultimately seeks to optimize cybersecurity operations. The aim of this paper is to contribute to the discussion of this new form of defence for our national power grids. Specifically, the challenges of deploying such technologies.
Machine Learning Methods
Machine learning can be defined as algorithms and processes (mathematical techniques) that generalise past data and experiences in order to predict future outcomes, allowing us to draw inferences from data(Chio & Freeman, 2018).
Whilst this study does not expand on the specific mechanisms of ML and how it works, it is worth noting some examples of different types of ML with examples of how they work. These are:
· Supervised learning: various algorithms generate a function that maps labelled inputs to desired outputs. Relying on labelled inputs and outputs for the training data to learn.
· Unsupervised learning: models a given set of inputs and returns patterns and classifications of the inputs without the reliance of labelled inputs and outputs.
· Semi-supervised learning: combines both labelled and unlabelled inputs to generate a function or classifier for the given inputs.
(G. Carbonell, S. Michalski, & M. M, 1983)
Literature Review
Introduction
This section presents an overview of the current CSDS landscape and the impact of cybersecurity attacks on countries financially but also within the context of their power grids. The discussion includes a brief history and analysis of CS policies which have influenced the field and looks at relevant studies associated with CSDS. Moreover, the limitations of the literature are considered.
Overview of Current CSDS Landscape
There are multiple threats within cybersecurity such as malware attacks, social engineering, phishing, unauthorised access, zero-day attack, and denial of service(Sarker, et al., 2020). The frequency of these events is only increasing due to the rise of IoT and reliance on digital services(Li, Da Xu, & Zhao, 2015). These are logical consequences of a transition to and reliance on digital services. Due to the variety, scale and profile of these incidents, a paradigm shift in defence techniques was needed from reactive detection to proactive prediction methods(Zhang, et al., 2018). Many data science techniques such as machine learning (ML), data mining (DM) and artificial intelligence (AI) are now being used within cybersecurity(Bechor & Jung, 2019). These techniques can be broken down into subcategories of uses:
1. Applying DS methods to literature/datasets to identify issues, opportunities, and CS challenges(Bechor & Jung, 2019),(Humayun, Jhanjhi, Talib, Shah, & Suseendran, 2021).
2. Applying DS techniques to CS in order to quantify cyber risks, and optimise cybersecurity operations(Sarker, et al., 2020).
With specific focus on subcategory 2 mentioned above, DS is transforming how CS approaches security methods like firewalls, authentication, access control and cryptography(Sarker, et al., 2020). It is important to mention that some of these traditional methods of protecting access to computer networks now may not be effective against modern cyber threats as the aforementioned methods only provide external security and are inadequate in detecting internal attacks and protecting a network(Anwar, et al., 2017). But combined with automated intrusion detections systems, these may provide better security for a computer network(Amine Ferrag, Maglaras, Moschoyiannis, & Janicke, 2020).
Machine Learning Techniques in Cybersecurity
Where data science and cybersecurity meet are within intrusion detection systems (IDS), which detect issues by analysing security data from core or weak points in a computer network(Brahmi, Brahmi, & Ben Yahia, 2015). However, this study will not delve into the different types of IDS’s but will instead focus on the automation of these systems. Sarker, et al.,(2020)suggests that automating these IDS’s could be more effective, because it does not involve a human interface between the detection and response systems. Instead, the IDS would utilise support vector machine, random forest, and multilayer perceptron classification methods to spot intrusions to the network traffic(Singh, Venter, & Adeyemi Ikuesan, 2022).
An example of such technology would be the Cylance anti-virus software from Blackberry. Cylance anti-virus software utilises ML techniques to detect intrusions on a device or network(Blackberry, 2023). However, researchers were able to bypass Cylance IDS by adding benign code strings from a video game into the malicious code, which showed that Cylance failed to detect almost 90% of 384 malware programs that were amended with video game code(Barth, 2023). Within the context of power grids this could have devastating impacts on a country’s security of critical infrastructure, especially when research has not shown significant evidence for a strong ML method to be applied specifically for IDS’s(Singh, Venter, & Adeyemi Ikuesan, 2022). Other examples include Darktraces’ Heal software(Darktrace, 2023)and Tessians’ cloud email security(Tessian, 2023)
Impact of Cybersecurity Attacks
As highlighted by Castillo(2014), the effects of power outages can include economic, social, and physical. This section will review the impacts of cybersecurity attacks on critical infrastructures such as financial markets, healthcare systems and power grids through these 3 lenses.
Physical Impact
Over the past decade the importance of CS has become as imperative as military or law enforcement security(Armerding, 2023). This is further evidenced by many examples, one being the experimental remote destruction of a large diesel power generator dubbed project Aurora conducted by the US Department of Energy’s Idaho lab. This was achieved by issuing supervisory control and data acquisition (SCADA) commands(Meserve, 2023). A much larger scale attack of this nature could cause physical damage to a country, power outages and personal data breaches on a scale hitherto undreamt of(European Commission, 2013). As mentioned previously with the increased use of IoT this inherits the potential harm of ransomware on government agencies, healthcare and transportation of nations utilising smart grids(Habibzadeh, H. Nussbaum, Anjomshoa, Kantarci, & Soyata, 2019).
Financial and Economic Impact
There is also significant evidence for the financial and economic impact of cybersecurity attacks. Targeted firms listed on stock exchanges can see losses of 1-5% in the following days of an attack(Cashell, D. Jackson, Jickling, & Webel, 2004). In some cases, causing a company to file for bankruptcy, as with Nortel networks a Canadian based telecommunications company in 2009 after Chinese hackers infiltrated their network(Kumar Venkatachary, Prasad, & Samikannu, 2017). It proves difficult to measure an accurate financial or economic cost to cyber-attacks as companies are reluctant to report their cyber-attacks. This created a gap in the data on their impact between internal data of organisations and publicly available data for research(Brian, William, Mark, & Baird, 2004). However, within the context of power grids a cyber-attack would have unequivocable impact on trade, competitiveness, innovation, economic growth and GDP for any firm or economy(Venkatachary, Prasad, & Samikannu, 2017).
Societal Impact
With electricity being defined as a vital satisfier of basic human needs, power outages can have profound impacts on communities(Brand-Correa & Steinberger, 2017). Societal impacts were demonstrated when the NHS was attacked in 2017 cancelling outpatient appointments due to their systems being shut down by a worm computer virus(Venkatachary, Prasad, & Samikannu, 2017). And again in 2022 when ransomware targeted patient data(Milmo, 2023). Countries like India and Pakistan, both lost industrial outputs caused by power outages. These events have reduced GDP by 1.5-2% (or potentially more as the measures of the reduction are static and single period)(P. Sanghvi, 1991). It has also been demonstrated that there has been food related, medical devices, physical and mental health impacts to society within power outages. Here, the main populations effected were children, English as second language speakers, rural populations, healthcare workers, racial and ethnic minorities(Andresen, Kurtz, Hondula, Meerow, & Gall, 2023).
Impacts on Power Grid
With a clear impact of cyber security attacks on physical property, societal services, financial and economic impact demonstrated by the literature, the table below created by (Venkatachary, Prasad, & Samikannu, 2017)details specifically the impacts of an attack on various elements of a power grid.
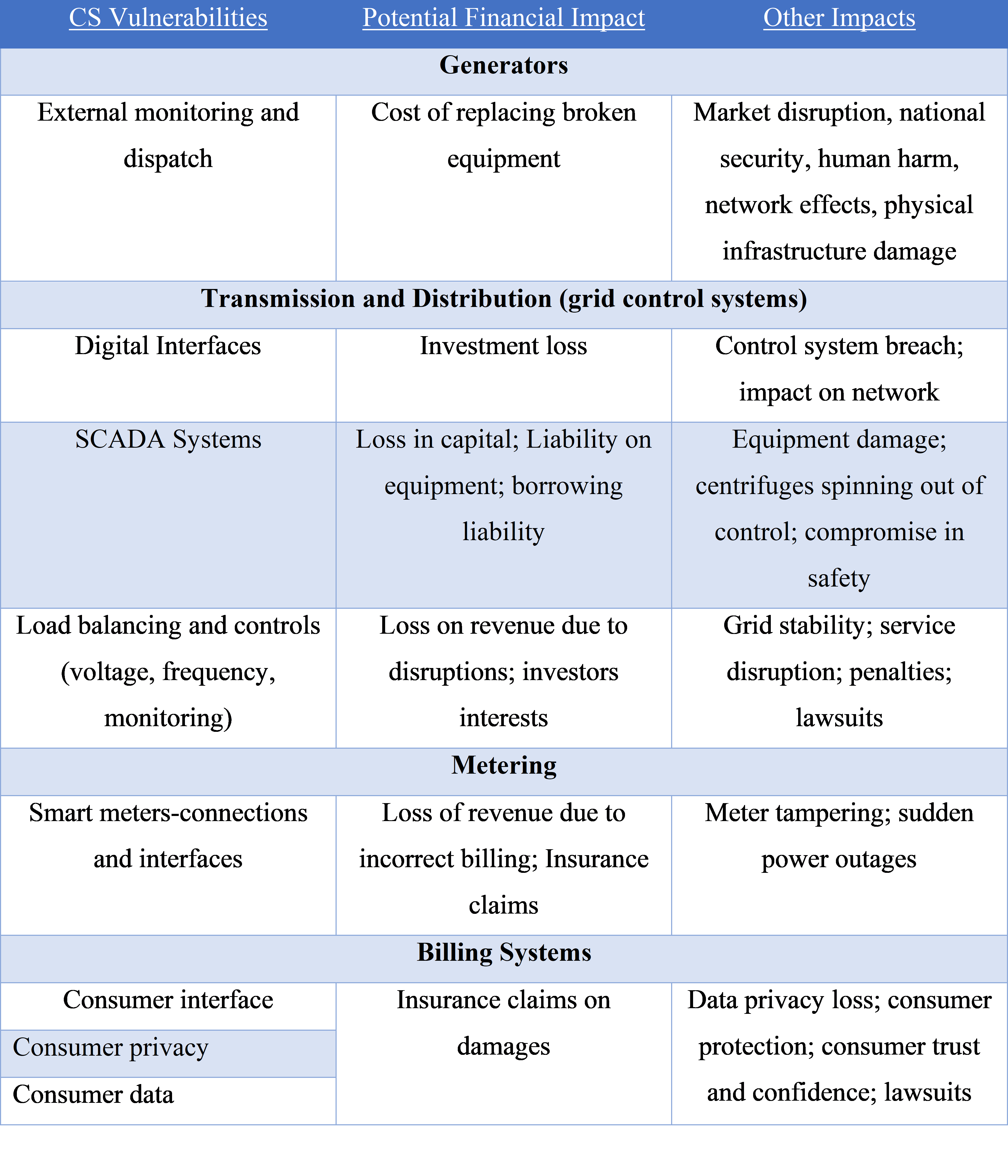
Highlighting the impacts of CS attacks on critical infrastructure it can be concluded it is in every country’s interest to invest into the CS of their infrastructure. As also recommended in the 2017 Energy Expert Cyber Security Platform (EESCP) report(EECSP, 2017).
Influential CS Policies
Within the European Union (EU) there have been several developments in their CS policies starting with the Cybersecurity Act enacted on 13/09/2018 where negotiations were held to reach agreements by member states on the act by the end of the year. The act was meant to enhance cyber resilience by providing an EU-wide certification framework for IT products, services and processes with this policy being implemented in April of 2019(European Union, 2023).
On 30th of July 2020, the EU council imposed the first ever sanctions against cyber-attacks against 6 individuals and 3 entities responsible for or involved with large scale cyber-attacks within Europe. This included a travel ban, asset freeze and a ban on any EU persons making funds available to these individuals(European Union, 2023). These restrictive measures were further extended until 18/05/2025 to protect the EU and its members states against significant cyber-attacks.
During May 2022 the EU Council and EU Parliament reached an agreement on the Digital Operational Resilience Act (DORA), which ensures financial sector firms in Europe can maintain operations throughout a severe operational disruption through a cyberattack(European Union, 2023). DORA essentially ensures that firms can withstand, respond to, and recover from all types of cyber threats and disruptions (European Union, 2023).
Previous CSDS Studies
A pivotal study in the research into CSDS was by (Sarker, et al., 2020) who provided a machine learning multi layered framework for the purpose of cybersecurity modelling. This framework detailed how it could be possible to discover security insights from the raw data to build smart cybersecurity systems, considering the machine learning techniques, incremental learning, and dynamism to keep the model up to date (see the framework below). This paper provided further research questions to be explored including establishing large trustworthy cybersecurity datasets to test ML techniques on and handling quality problems within the datasets.
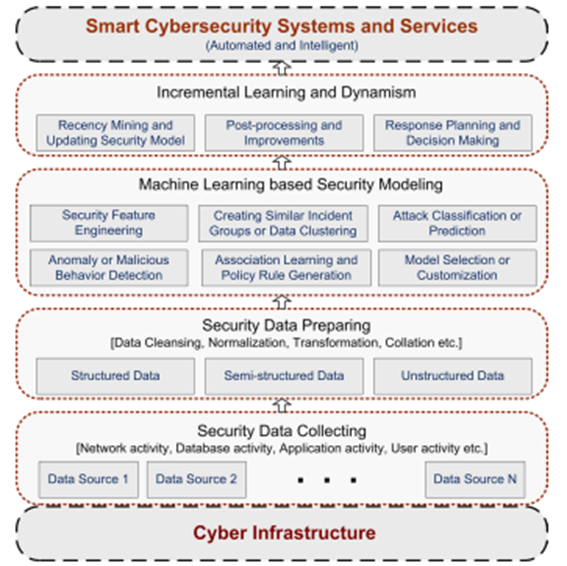
There have been several studies observing ML methods being applied to cybersecurity practices concerning power grids and that follow the framework proposed above.(Buczak & Guven, 2016)conducted a study on ML approaches used by IDS’s. Acknowledging the importance of datasets for the training of ML models for IDS’s, they classified the observed datasets into three types: packet-level data, NetFlow data and public datasets. Stating it is difficult and time consuming to achieve representative data sets for the threat an organisation may want to detect in their system, but also that there is a lack in the available labelled datasets for the ML algorithms to learn from(Buczak & Guven, 2016). Unfortunately, the ML methods for cyber security applications were not established in Buczak & Guvens’(2016)study and due to the complexity of each method it is hard to make a recommendation for the type of attack the ML system is supposed to detect.
Zarpelao, Miani, Kawakani, & de Alvarenga(2017)provided a study on how these intrusion detection approaches would apply to the IoT. The study classified IDS’s based on the detection technique, IDS placement technique, and security threat. The study found that these methods are still in early stages of development and there is no clear consensus on the most suitable detection methods even though they exist. Also, similar to the results from(Buczak & Guven, 2016)stating that there were no suitable labelled data sets for validation of the models.
Limitations in Literature
Validity of CS Datasets
As stated by Buczak & Guven(2016), it is difficult and time consuming to collect representative data of the exact intrusion scenario you are trying to train an ML model for. Also, as stated previously by Brian, William, Mark, & Baird(2004), companies are reluctant to report their cyber-attacks and this has contributed to unrepresentative data on the number of attacks, type, origin, impact etc.
Validation of ML Methods
Throughout the literature of ML methods being applied to IDS’s there are no labelled datasets to measure the accuracy and validity of the ML model against(Buczak & Guven, 2016). This makes it hard for researchers to suggest an appropriate model for IDS’s to utilise. The models have demonstrated that they work in detecting intrusions in networks, but which method is most appropriate is inconclusive(Zarpelao, Miani, Kawakani, & de Alvarenga, 2017).
Foreseeing Challenges of Implementing ML Methods
Most studies reviewed in this literature review are highly technical, detailing methods for ML methods to be applied to IDS’s of power grids and trying to assess the validity of the models, but none of the studies reviewed consider the challenges of having such algorithms controlling part of the defence mechanisms for one of our most crucial critical infrastructures.
Conclusion
Given the intricate nature of protecting these critical infrastructures and the potential devastation caused in failing to do so – we need to implement DS methods with extreme care, the risks of this have already been demonstrated when financial and healthcare services have experienced cyber-attacks. This study acknowledges that complete automation may not be possible(Sarkar, Teo Meng, & Chang, 2022)but it is imperative to identify the implications if something were to go wrong.
Methodology
Research Philosophy
Imprecisely formulating and stating theories can obstruct empirical testing and the results of studies conducted(Astley & Van de Ven, 1983). Therefore, it is crucial to accurately represent data collected and supporting of the research aims and objectives.
This research embodied the critical realist philosophy presented by(Miller & Tsang, 2010), the theory suggests that empirical data (such as a policy review and interviews) can support the critical evaluation and creation of theories. This was chosen over the falsification research philosophy as suggested by Popper(1959)which aims to test and falsify existing claims that are never certain and definitive(Sayer, 2000). Also, this approach is more suited to sociotechnical topics as it allows the researcher to understand the underlying social mechanisms of the research by observing realistic data(Buchanan & Bryman, 2009).
Research Approach
This study employed a qualitative, inductive research method. This involved creating codes from the policy documents and interview transcripts through thematic analysis, which was chosen because there is no established code framework to follow for a deductive approach. Moreover, thematic analysis was chosen as it provided the study flexibility to derive new themes from the qualitative data retrieved, allowing for the discover of new information from qualitive research(Braun & Clarke, 2006). Acknowledging the critique of Antak, Billig, Edwards, & Potter(2002)in that “anything goes” in thematic analysis allowing for potential researcher bias, interpretation of themes, and a non-limited constrained creative aspect to this method. Thematic analysis was still chosen as the flexibility of following the methodology as proposed by Braun & Clarke(2006)ensured the method is theoretically and methodologically sound whilst maintaining its flexibility which allows for new insight to be gained.
Methodology Overview
The literature and policy review were essential to first identify the key issues that related to cybersecurity of national power grids and how data science can help with combatting these issues. To further contribute to this data collection, semi structured interviews provided fresh expert knowledge from professionals in the field supplementing the information gathered in the policy review. Ethical standards were maintained throughout this process.
Data Collection
Policy Review
The policy review deployed thematic analysis to derive insight from the policy documents retrieved from the Overton database. As defined by Scott(1990)a policy is an artefact which has inscribed text as its central feature. With the “naturalistic and unobtrusive nature” of documents during document analysis the data is found as opposed to being made through other forms of analysis(Jensen , 2002), this methodology precisely aligns with the aforementioned critical realist research philosophy of observing the information that is to hand.
There are several advantages of document analysis including: efficiency (document analysis is less time consuming and requires data selection rather than collection), with this efficiency also brings cost effectiveness (collection of new data on the topic of cybersecurity methods of national power grids would not only be costly but also a political subject to breach and would most certainly be a close guarded secret of governments and grid operators), and stability (the investigators presence does not alter the state of the documents being studied)(Bowen, 2009). This stability could be challenged with the choice of thematic analysis on the documents in which the researchers bias could distort their interpretations of themes derived as highlighted by Antak, Billig, Edwards, & Potter(2002). However as discussed, the flexibility of this method will aid this study to uncover new knowledge(Braun & Clarke, 2006).
As stated above the Overton database will be used to collect 8 copies of policy documents selected by the following filters in the search:
· Keywords: "cybersecurity","power grid","energy","data science"
· Location/Source Country: USA
· Topics: “Smart Grid”
Of the 20 policy documents returned by Overton, 8 were selected based on the frequency of the keywords searched appearing in the documents. Some documents returned, only included mentions of power grid and cyber security, this study aimed to observe documents including at least the 3 keywords from the search “cybersecurity”, “power grid”, “data science”. Documents from the USA only were selected as the USA has experienced the most financial impact from CS attacks in recent years, but also the highest share of networks affected from global attacks (such as the Stuxnet infection)(Venkatachary, Prasad, & Samikannu, 2017). These will be exported as .pdf files and stored for further data processing. The policy documents collected were as follows:
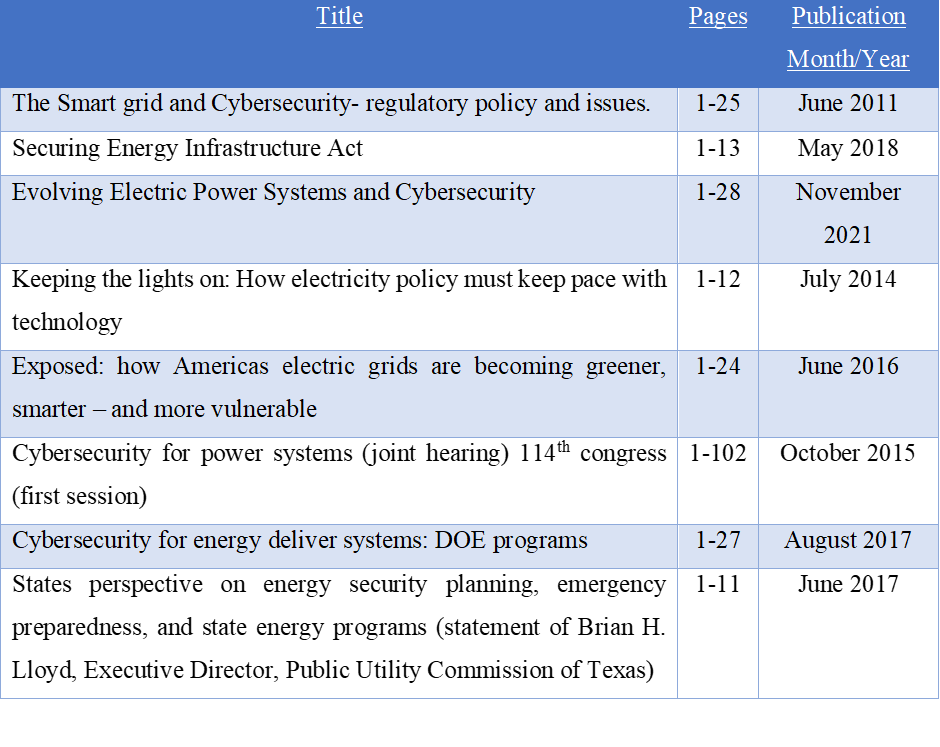
Interviews
This part of the data collection utilised online, semi structured, interviews with two professionals who both work in the cybersecurity industry. Despite interviews being a resource demanding data collection method in terms of planning, conducting, and analysing the results(Hove & Anda, 2005), this method was chosen as the third research objective of this study was to further contribute to the discussion of DS practices being applied to CS. Choosing this data collection method allows for autonomy to explore new ideas whilst still giving structure for the interviewer(A. Adeoye-Olatunde & Olenik, 2021).
Prepared questions will give the interviews a structure, with notice of the question topics given to participants on an information sheet upon contacting them to partake in the study. The following questions were chosen as they provided a structure for the semi structured interviews to contribute knowledge to the research aim and objectives. The questions were as follows:
Interview Questions
1) What is your role, professional background, and sector you work in?
2) What is your definition of the terms Data Science and Data Science methods?
3) What sort of developments have you personally seen in using Data Science methods for cybersecurity of critical infrastructure i.e., power grids or other? If any?
4) What benefits or opportunities do you think there are when using Data Science methods within cyber security practices?
5) What do you think are the disadvantages of using Data Science methods (machine learning) within cybersecurity compared to using humans in similar roles?
6) Do you think there are any risks of using such methods in cybersecurity, to either society, companies, or governments? If so, can you tell me more about these risks?
7) What policies should be considered with regards to the implementation of Data Science methods (machine learning) within the cybersecurity Industry?
8) Where do you see key future developments within the cybersecurity industry (with relation to the implementation of Data Science methods)?
Interviews were organised at a convenient time on google meets, with the permission of the participant and transcribed via the Tactiq chrome add on software, which has been approved by the University of Sheffield for transcription of low-risk projects like this study. This study conducted 2 interviews for the thematic analysis this number was chose to focus on the individual’s unique perspective instead of a collective (A. Adeoye-Olatunde & Olenik, 2021). Each interview was given an hour maximum, conducted as a conversation with one interviewee at a time. This is done as to reduce fatigue for both the interviewer and respondent during the interview as suggested by Adams(2015). An advantage of this method is having an interviewer, in this case, the researcher, present to clear out any queries raised during the interview. However, with a structured interview there is no opportunity to delve deeper into points raised by the participant(Cachia & Millward, 2011)therefore further enriching the information provided by utilising semi structured interviews. It is noted that a disadvantage of in person semi structured interviews is that the participant can feel exposed in a public setting when sharing their point of view(Sturges & Hanrahan, 2004), however this was negated by conducting the interview in an online meeting which allowed the participant to pick an environment they are comfortable with.
Sampling
For the interviews, non-probability convenience sampling was used to select professionals. This was done to make sure that a breadth of individuals qualified for interview participation in line with the logic of the study(Punch, 2004). The only requirement is that the participant work in either or a combination of a role in relating to data science/cybersecurity/national power grids. This sampling method was chosen considering the time and resource limitations of this study. Participants were sourced through the professional social media website linkedin.com. Filtering members with the search bar via keywords such as: “cybersecurity”, “penetration tester”, “national power grid”. Members will then be selected based on their displayed job title.
Data Analysis
Coding
Coding is defined as a method performed iteratively to find keywords within qualitative data based on relevance, frequency, and that supports the research question(Yoonsang, Huang, & Emery, 2016). Additionally, coding was deployed by the researcher by reading the 8 documents collected. A coding book will not be created by the researcher due to the time constraints of this study as the process of coding is already difficult and time consuming (Suhr, Cutrona, Krebs, & Jensen, 2004). There are also no known existing coding frameworks at the time of this study for this type of policy analysis. As no statistical analysis will be conducted during this study codes belonging to multiple themes was allowed for each unit of coding, if done whilst conducting content analysis this can impact the results of statistical analysis.
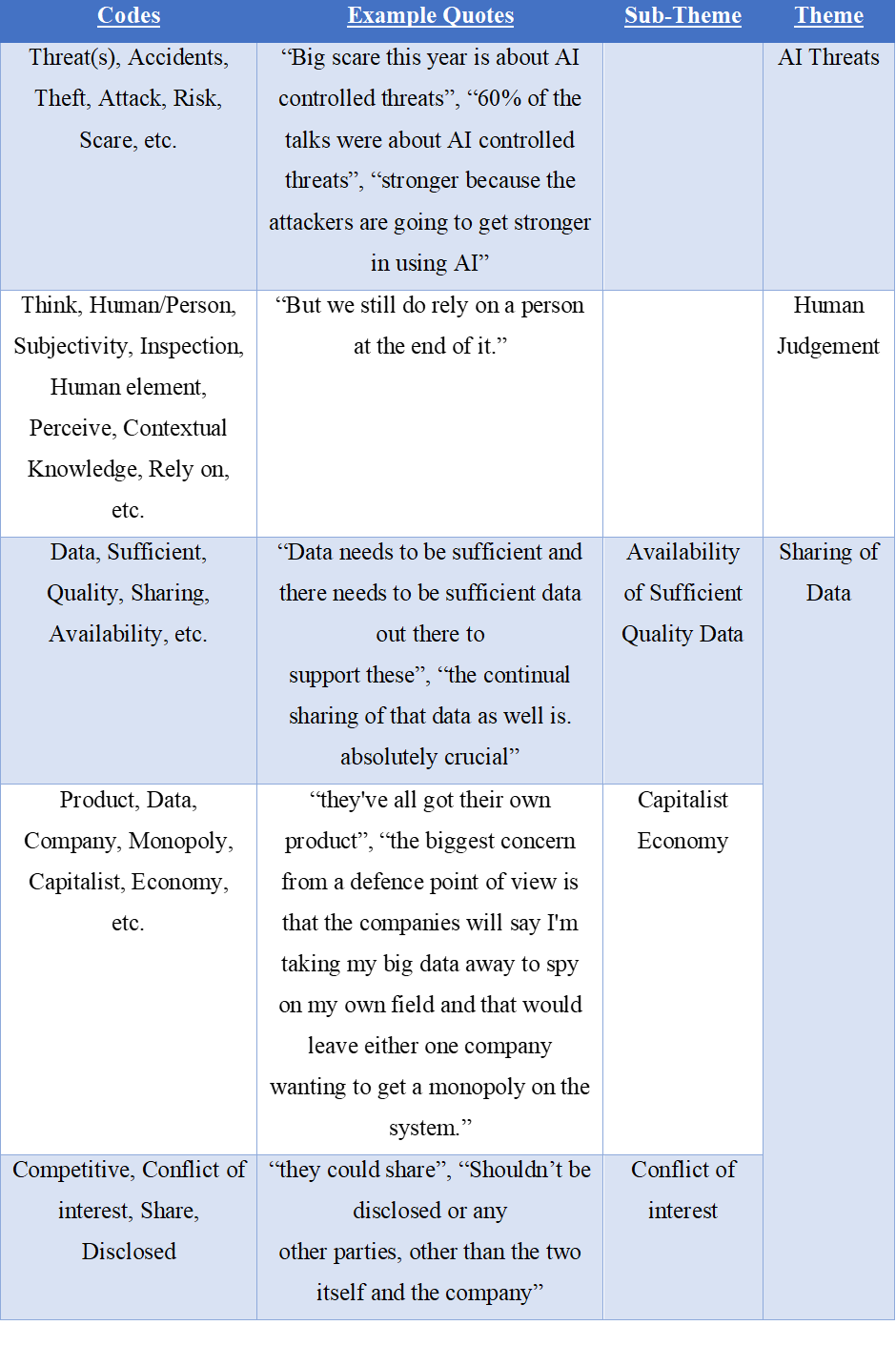
Thematic Analysis
For the data analysis of the policies collected a thematic analysis approach has been chosen as the data produced by this study will mostly be qualitative (interview transcripts and policy documents). This approach was appropriate for systematically identifying, organizing, and offering insight into patterns of meaning (themes) across a data set(Braun & Clarke, 2012). Although content and thematic analysis are similar, thematic analysis was chosen because this methodology is known to provide depth to the meanings of the code(Braun & Clarke, 2012)which would be required for the third research objective of contributing knowledge. Therefore, choosing content analysis for the policy review could potentially limit the insight of this study. Thematic analysis is more appropriate for developing reasons behind qualitative data with less of a focus on statistics (Vaismoradi, Turunen, & Bondas, 2013), this study aims to investigate current challenges of data science being applied to cyber security practices of national power grids.
To perform the thematic analysis the interview transcripts and relevant policy documents were uploaded to NVivo software for analysis and to identify concepts and ideas relating to the research question. NVivo was used to apply a manually produced code to identify themes and sentiments in the documents. Once the documents had been analysed, themes of challenges and common occurrences of themes were selected to identify the relevant challenges of data science being applied to cybersecurity practices of national power grids.
Resource Requirements and Constraints
Overton Database
The policy review utilised the Overton Policy Database (https://www.overton.io/). Overton was chosen because it includes a selection of worldwide relevant official documents and policy information about the cybersecurity of national power grids. The University of Sheffield provides a free subscription for all their students which was utilised throughout this study. However, the database is not publicly accessible. Instead, it requires a subscription for universities (priced by research intensity), think tanks, international government organisations and agencies ($1,800-$7,200). A free trial is available after providing contact and organisational details which is then reviewed by Overton but there are restraints on accessing the full version of this database as a member of the public. The previous points would need to be considered for anyone replicating this study.
NVivo Pro 1.6.1
For the thematic analysis of the policy documents downloaded from the Overton database the NVivo Pro software version 1.6.1 was utilised, created by Lumivero. This software was chosen as it assists researchers collect, manage, visualise, and report their qualitative data systematically and individually(Dhakal, 2022).
Interviewing Industry Professionals
The interviews of professionals required access to preliminary contact information of these professionals available via the professional social media site LinkedIn. Access to a computer to host the virtual interview, which will be held on google meet (requiring an email to access interview), recording software (provided within google meet) and transcription software (Tactiq a transcription add on for google chrome compatible with google meet) on the device. Accessing the contact information for these individuals may be hard to replicate for researchers with no ties to industry or contacts.
Limitations
The first limitation to consider if this methodology was repeated would be the accessibility of the Overton database to retrieve the policy documents as mentioned the database is not publicly accessible requiring a subscription, the price of which can range from $1,800-$7,200.
No coding book is used during the study and nor will one be developed through inter-rater reliability (where two or more independent raters code the same data) with particular attention paid to how these results are distinguishable from each other(Chen, Gisev, & Bell, 2013). This was due to the resource constraints of this study and not being able to employ another coder to perform this method, given it was an assessed piece of coursework. However, in a different context with more time and resource allocation it would be recommended to perform this to ensure more reliability of the themes deducted from codes identified(Chen, Gisev, & Bell, 2013).
With the main methodology and themes coming from document analysis there are some disadvantages of this method that need to be considered such as: Insufficient detail (The documents are produced for uses other than research created independently of research agendas, which may not provide sufficient detail to answer the research question), Difficulty retrieving (Policy documentation is sometimes not retrievable, with some policy documents deliberately blocked from the public eye due to concerns with national security in this studies case), and Biased selectivity (Documents are likely to be aligned with corporate policies and procedures and with the interests of organisations in mind) (Bowen, 2009).
Ethics
This study has received approval and a risk category of “Low Risk” from the University of Sheffield and upon review from the dissertation supervisor has been risk categorised as low. The study involved human participants. However, none are considered vulnerable, and no sensitive topics would be identified. Rather the discussion focused on professional topics like their experiences with DS methods being applied to CS. Participants received an information sheet and email outlining the research content, interview questions and providing a consent form to be signed. Verbal consent for the commencement and recording of the interview was also asked at the start of each interview.
Identifying information of names of participants or institutions during the interviews of this study were anonymised from the study and redacted if mentioned in the recording. Political views and sensitive information were not discussed. However, this data will not be needed for the purpose of the study and hence its low-risk categorisation. Upon completion of the MSc in Data Science course any interview transcripts or recordings will be deleted. As identified by Reilly(2014) re-identification of the interviewees may be possible if too much information of their political or personal identity is revealed in the interview, however the interview questions were designed in such a way as to not ask about any personal information and keep the questions topics around CSDS.
Results
Thematic analysis(Braun & Clarke, 2012)of the policy documents chosen and interview transcripts revealed 4 main themes of discussion: Critical Infrastructures, Cybersecurity Organisations, Cybersecurity Technique(s), and Data Science Methods.
Two sub-themes were identified within the data science methods theme: the Advantages of ML relating to the negation of human errors and Disadvantages of ML methods. The latter sub-theme revealed a plethora of further sub-themes including AI threats (with a further sub-theme of reactive defence), Human Judgement (with a further sub-theme of the breadth of data science), Lack of Policy (with sub-themes of Hesitation of using AI and the NIS Directive), Sharing of Data (with sub-themes of the availability of sufficient quality data, the capitalist economy, and conflicts of interest) and Time Sensitivity.
These sub-themes specifically identified challenges with the adoption of data science methodologies within CS practices of critical infrastructures similar to or relating to national power grids, which answers the research question “What are the challenges with the adoption of Data Science within cybersecurity practices of national power grids?”. Further analysis conducted of these sub-themes in section 5 addressed the research aim of investigating the “challenges, benefits and consequences of the application of data science methods to cybersecurity practices of national power grids”. Ergo, helping to achieve the research objectives 2 and 3 (see section 1.2.2).
The following section 4.1 will present the results of the thematic analysis of the policy documents and interviews detailing specifically the 5 sub-themes found under the sub-theme of Disadvantages of ML as they directly support and are relevant to the research question, aim and objectives. This section will display summative statistics of the sub-themes, whilst also going into detail of the sub-themes outlining what they entail, the scope of the theme, sample extracts, and any links or contrasts to other themes.
Section 4.2 will summarise key findings from the interview transcripts to help achieve research objective 3 (please see section 1.2.2) and further enrich the discussion, summarising key quotes and comparing the responses from the two cybersecurity professionals interviewed.
Section 4.3 provides a summary of the key findings from the thematic analysis of policies and interviews. In particular, it looks at how these relate to the research question and aims of the study. It also considers the usefulness of all themes identified and if any gaps exist which need to be considered within the data analysis.
Thematic Analysis of Policy Documents and Interviews
This section will present the results of the thematic analysis of the policy documents and interviews, specifically exploring the sub-themes found within the theme of Disadvantages of ML as they are most relevant in answering the research question and supporting the research aims. Figure 5 details the structure of the various themes and sub-themes identified within the thematic analysis.
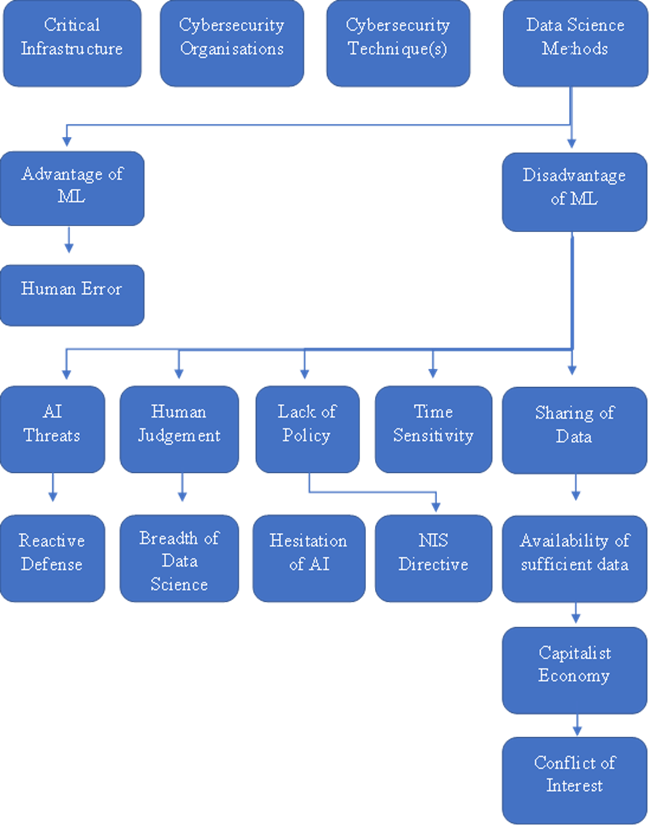
Summative Statistics of Themes by Policy Document
For the sake of time I won't transform every table for the summative statistics in to images to then import into the ghost portal however if you are interested you can get in touch with me for a copy of them.
Most of the significant results are discussed in the text following.
Theme 1: AI Threats
The sub-theme of AI threats entailed anything relating to AI that could be deemed a threat to cybersecurity of a national power grid or a threat posed by AI that could contribute to, or be involved in. Key words or phrases used in the context of AI were deemed suitable for this theme such as: Threat(s), Accidents, Theft, Attack, Risk, AI, etc. The sub-theme did not include any discussion or mention of AI in a positive or neutral way.
Sample extracts of the sub-theme:
· “Absolutely. I mean the big scare this year is about AI controlled threats.”(Participant 1, 2023)
· “Now the U.S. faces a new risk—cyberattacks—that could threaten public safety and greatly disrupt daily life.”(Mills, 2016)
· “Attackers are relying on AI to modify code to get through the current defences, the more, but that we're gonna have the industry is going to have to rely on AI, to react in a speedy fashion to be able to defend, or to be able to prevent intrusions.” (Participant 1, 2023)
The sub-theme was observed in every document analysed other than “The Smart grid and Cybersecurity - regulatory policy” with the most mentions in the interview transcript with participant 1 (N=13). Throughout the thematic analysis, AI Threats was mentioned 22 times in the policy documents and 15 times in both interview transcripts, a total of 37 occurrences. Thus, AI threats accounted for 23% of the sub-themes discovered within the sub-theme of the disadvantages of ML. This is obviously a persistent and well recognised issue by both the professional interviews and policy documents unlike other themes. Unlike the theme of human error which details a more positive side to the use of AI and ML in the context of using such methods to negate human errors in cybersecurity.
Theme 2: Human Judgement
The sub-theme of human judgement included any words or phrases associated with human oversight of AI being needed or in the context of AI lacking that human judgment/contextual knowledge of the data being analysed. Key words or phrases deemed suitable for this theme included: Think, Human, Subjectivity, Inspection, Human element, Perceive, Contextual Knowledge, etc. This sub-theme did not include comments, words or phrases that could be classed as a human judging something not relevant to AI. Instead, the theme included human judgment as a component to the AI/ML methods process and not human judgement like an opinion. Throughout the thematic analysis, Human Judgement was mentioned 3 times in the policy documents and 17 times in both interview transcripts, a total of 20 occurrences, accounting for 12% of the sub-themes discovered within the sub-theme of the disadvantages of ML.
Sample extracts of the sub-theme:
· “So, I would never want to remove… The human element, the human to be able to make and to override”(Participant 1, 2023)
· “As SCADA technology has matured, system control has become more intelligent and more automated, requiring less human intervention.”(Congressional Research Service, 2017)
· “Rather than resting the success of our cybersecurity efforts on programs that require changes in human behaviour, we might have better success if we change our technology and processes to fit the behaviour of people.” (United States Congress, 2015)
This theme was observed mostly in the participant interview transcripts (N=13, N=4) with only 3 occurrences of the theme in 2 of the 8 policy documents analysed. This highlights a gap in the policy documents analysed of the human judgement required alongside the implementation of AI. There was a contradicting relationship between the human judgement sub-theme and human error sub-theme (which explored the benefits of AI/ML methods removing potential human errors from CS methods), as both interview transcripts revealed both themes of needing ML methods to negate human errors within CS systems and to improve time efficiency. Also, this underscored the need for that human judgement within these automated CS IDS’s, which will be discussed further in Section 5.
Theme 3: Lack of Policy
The sub-theme of Lack of Policy included anything relating to AI policy and the potential lack of it, or if there was mention that a future need exists. This includes mentions of future policies needed and direct criticisms of lack of current policy or attempts. Key words or phrases used in the sub-theme of lack of policy included: Lack, not enough, rules, can’t keep up, etc. The sub-theme did not include any discussion or mention of policy in a neutral way.
Sample extracts of the sub-theme:
· “it's all gone too fast like they can't keep up with the progression that it makes (referring to policies and rules around AI” (Participant 2, 2023)
· “brought up the lack of an approved products list that vendors such as myself or these smaller electric utilities can go to that has standards”(United States Congress, 2015)
· “… there are no policies. (When asked about what policies surround AI and ML methods in CS industry)”(Participant 2, 2023)
Throughout the thematic analysis a lack of policy was mentioned N=16 times in the policy documents and N=6 times in both interview transcripts, with all of the occurrences happening in the participant 2 transcript (N=6). A total of N=22 occurrences, accounting for 15% of the sub-themes discovered within the sub-theme of the disadvantages of ML. Here, there is clear evidence that there is an acknowledged lack of policy from industry professionals and policy makers.
Theme 4: Sharing of Data
The sub-theme Sharing of Data included anything relating to the dispersion of data whether in the context of collaboration for the purpose of enhancing CS practices, the potential conflict of interest arising from it, the contribution of capitalism to that conflict of interest, and any mention of data being shared for the practice of CS. This sub-theme was then divided into 3 more sub-themes pertaining to the contextual areas/themes discovered availability of sufficient quality data, Capitalist Economy, and Conflict of Interest. Key words or phrases used in the sub-theme were Data, Sufficient, Quality, Sharing, Availability, etc. The sub-theme did not include any discussion or mention of policies relating to the sharing of data or privacy laws. The theme was strictly within the context of private entities sharing data to form better CS practices.
Sample extracts of the sub-theme:
· “If they shut down, getting information from each other, don't give their data. The people for looking at these the vulnerabilities are manufacturers of the security products and so the, the firewall vendors, the antivirus vendors, The malware vendors. They need to play together realistically?” (Participant 1, 2023)
· Researcher: “Do you think that sort of competitive conflict of interest between firms could halt that collaborative effort?” Participant 1: “I do.” (Participant 1, 2023)
· “leveraging government capabilities to gather intelligence on threats and vulnerabilities, and share actionable intelligence with energy owners and operators in a timely manner” (Murkowski, 2018)
Throughout the thematic analysis the sharing of data was mentioned 19 times in the participant 1 interview transcript and 13 times in the participant 2 transcript. However, this theme was not mentioned in the policy documents. A total of 32 occurrences, accounting for 20% of the sub-themes discovered within the sub-theme of the disadvantages of ML. This establishes clear evidence that experts in CS are ruminating the ideas of the impacts of sharing attack data with other companies to make the industry more secure.
Theme 5: Time Sensitivity
The sub-theme Time Sensitivity included anything relating to the time in terms of reaction of a system breach, reaction times in AI vs humans, but also any mention of the timeline of policy. Key words or phrases used in the sub-theme were response time, time, expire, slow, quicker, speed of reaction etc. The sub-theme did not include any discussion or mention of future predictions or past references as a mention of time or stating the time of day of a particular senate meeting/policy document.
Sample extracts of the sub-theme:
· “At INL, industry can test control systems technology in real world conditions, reducing response time and risk for future attacks.” (United States Congress, 2015)
· “Run millions of iterations of analysis, analyses. So, the biggest the biggest benefit realistically is speed and time.” (Participant 1, 2023)
· “Idaho National Laboratory is providing a cyber-incident response comparison capability and enabling industry to work towards an automated response capability to a cyber-incident and measuring the efficacy of automated response to drive future improvements”(Murkowski, 2018)
Time Sensitivity was mentioned 24 times in the participant 1 interview transcript and 5 times in the participant 2 transcript. And a total of 19 times in the policy documents . A total of 48 occurrences, the most mentioned theme in the thematic analysis, accounting for 30% of the sub-themes discovered within the disadvantages of ML. This establishes clear evidence that time is an important factor in addressing the challenges facing the implementation of AI/ML methods to CS, whether that be the human vs machine reaction time, reaction to a system breach, and the timescales in which these new technologies are growing.
Summary of Key Findings
The thematic analysis of the policy documents and interview transcripts unveiled 4 themes amongst the documents analysed: Critical Infrastructures, Cybersecurity Organisations, Cybersecurity Technique(s), and Data Science Methods. Of these themes Data science methods had the most references (N=32).
Within the data science methods theme, the sub-theme of Disadvantages of ML revealed 5 more sub-themes that revealed 5 challenges in the adoption of data science methods to cybersecurity practices within critical infrastructure and national power grids (AI threats, Human Judgement, Lack of Policy, Sharing of Data, Time Sensitivity). This contributed to achieving the research aim of investigating the challenges. Of these, time sensitivity (N=48), sharing of data (N=32) and AI threats (N=37) were the most mentioned themes.
Key findings relating to the sub-themes included:
· AI threats was observed across every document analysed.
· Human judgement occurred most in the participant interview transcripts (N=17), with only 3 occurrences in 2 out of the 8 policy documents reviewed.
· Lack of policy was identified more times in the policy documents (N=16) compared to the participant interview transcripts (N=6).
· Sharing of data was the second most common sub-theme identified (N=32), with no mention in the policy documents analysed.
Time sensitivity was the most common sub-theme found in the analysis, occurring in 5 out of 8 policy documents and in both interview transcripts for a total of N=48.
Discussion
The research aim of this study was to investigate the challenges, benefits, and consequences arising from the adoption of data science methods within cybersecurity practices of national power grids.
The thematic analysis results of policy documents and interview transcripts revealed 5 common sub-themes, which highlighted 5 challenges that related to the adoption of DS methods (AI, ML) in CS practices which fulfilled research objectives 2 and 3. These themes will be discussed in the following sections.
AI Threats
A prevalent sub-theme emerged across both policy documents and interview transcripts of AI as a threat to society when implemented within CS practices of critical infrastructures. The theme consisted of the threat of AI systems used for defence not detecting anomalies(Participant 2, 2023), or AI systems used for offensive capabilities modifying code to adapt to current defence measures(Participant 1, 2023).
As identified by Zhang, et al.(2018)(see section 2), defence techniques needed to evolve from reactive detection to proactive prediction with the help of AI and ML methods. Within an IDS utilising anomaly-based detections, a variety of ML methods can be deployed, such as random forest currently being suggested as the most accurate (99.84%)(Goel, Guleria, & Narayan Panda, 2022). However, Goel, Guleria, & Narayan Panda(2022)recognised that, in their study, results may not apply to real life due to imbalanced datasets, IDS systems cannot process these large volumes of data, and they may not respond accurately to new threats. This was identified in section 2.6.2 where Buczak & Guven(2016)questioned the validity and accuracy of these models due to a lack of labelled data sets. Furthermore, this was recognised in the interview transcript from participant 2 stating “it's a good concept, but the disadvantage of course, is that like, exceptions, you know, sometimes things happen for a reason, the machine learning will not know that.”. This raised concerns in the context of this study, because if such methods were deployed to protect a power grid, then the debatable 0.16% of threats not caught in the IDS may pose devastating impacts for a society reliant on electricity. Since this number may heavily be underestimated.
These errors in detection with ML may be exacerbated by the evolving offensive AI measures being used to adapt code to bypass an IDS. AI has the potential to exploit unknown vulnerabilities within IDS by utilising large data sets and neural networks to make predictions and adapt better to IDS surpassing ML methods(Whitlock, 2023). This is further evidenced in the thematic analysis with the interview transcript of participant 1, with 13 occurrences of the theme stating, “Attackers are relying on AI to modify code to get through the current defences… the industry is going to have to rely on AI, to react in a speedy fashion to be able to defend, or to be able to prevent intrusions”.
This evidence presents two challenges that contribute to answering the research question:
· The questionable accuracy of ML methods within an IDS to detect an adversarial AI threat, considering the consequences of an anomaly.
· The threat posed by advances of AI adapted code surpassing current defence mechanisms.
Human Judgement
This sub-theme was identified mostly in participant interview transcripts (N=17) and occurred in only 2 out of the 8 policy documents reviewed, with a low occurrence in the documents the theme did appear (N=3). This theme, extracted from the policy documents, and interview transcripts revealed a double-edged sword. One was the need for AI to mitigate errors in human judgement within IDS. The other was that DS methods need to be overseen by a human who has contextual knowledge and situational awareness to provide oversight to ML and AI defence methods.
Within the interview transcript of participant 1, it was mentioned that human judgment causes the greatest number of errors within the industry. Hence the need for AI and ML methods to mitigate these errors. The participant referenced Gartner(2023)who stated that by 2025 human failure will be responsible for over 50% of significant cyber incidents. However, where Gartner (2023)may be wrong is that similar rates of error were already observed 27 years ago, albeit not in the CS industry but in US nuclear power plant activities. There, 48% of 180 significant nuclear safety related accidents being attributed to human error(Marsden, 1996). Additionally, participant 1 also stated, “at the moment… human element (is) required.” and “But we still do rely on a person at the end of it”. This, therefore, reveals a paradox. For instance, there is solid evidence about the error inherent to human judgement, but at the same time industry professionals stress the importance of such judgement, because the risks of pure automation are, by contemporary standards, presumably higher than if practice were to be automated alongside a human specialist. This evidence within the sub-theme and existing literature contributed to the research aim of investigating benefits of the application of DS methods to CS practices of national power grids but fails to address the research question to identify the challenges with the adoption of DS to national power grid CS practices. However, the disparity in the occurrence of this theme within policy documents and interviews with professionals highlighted a clear lack of connection between industry and policy makers when considering the human judgement required alongside the implementation of AI to mitigate the errors of human judgement. This contributed partially to evidence that supports the next theme identified.
Lack of Policy
The lack of policy sub-theme was observed in one of the interview transcripts of participant 2 (N=6) and policy documents (N=16). The results acknowledged the ever-present gap between reactive policies keeping up with technological advancements. It has been shown in the literature, for example, that it is difficult for policy makers to keep up with new technologies(L. Bayuk, et al., 2012), partially due to the aforementioned rapid curve of progress in technological evolution now exceeding the rate suggested by Moore's Law (Perrault, 2019). It is clearly recognised both by policy makers and professionals that there is a lack of policy or none at all around the practices of AI. This was shown in the transcript when participant 2 stated that “it's all gone too fast like they can't keep up with the progression that it makes (referring to policies and rules around AI)”.
AI is developing faster than policy can keep up with(Patanakul & K. Pinto, 2014), which harbours some benefits for the innovation of national power grids. But this rapid innovation contributes to an ever-expanding gap of knowledge between industry and policy makers as demonstrated in the results of this theme. This is made worse, perhaps, by the lack of industry-wide standards around the regulation of AI(Whitlock, 2023). This presents a third challenge with the adoption of data science methods, contributing to answering the research question:
· The balance between technological innovation and policies overseeing these new technologies.
Sharing of Data
Studies presented in the literature review gave credence to the lack of available labelled datasets for ML algorithms to learn from(Buczak & Guven, 2016)and also validation methods for techniques implemented(Zarpelao, Miani, Kawakani, & de Alvarenga, 2017). Fuelled by private companies reluctance to report their cyber-attacks(Brian, William, Mark, & Baird, 2004), as highlighted in the literature review, has only contributed to this lack of data. It has been suggested by Paul Nicholas and Cristin Flynn Goodwin(2015)in their time at Microsoft that the sharing of cybersecurity data could help the CS profession adapt their IDS systems and threat prevention measures; this solution was echoed in the thematic analysis conducted in this study. With the sub-theme occurring 32 times only in participant interview transcripts, this clearly identifies a lack of consideration if any from policy documents on this matter. Within this sub-theme, 3 more sub-themes occurred: availability of sufficient quality data, capitalist economy, and conflict of interest. These results evidence that industry professionals and academics alike are aware of the lack of data that could prove beneficial for the CS industry.
It has been observed that private firms are less likely to collaborate by sharing data specifically within CS, according to a meta-analysis of 82 papers conducted by Pala & Zhuang(2019). However, the meta-analysis does not reveal the cause of this reluctance to share data, a section observed in the interview transcript of participant 1 may suggest a reason for this:
Researcher: “… being in sort of a capitalist economy that we're in now. Do you think that sort of competitive conflict of interest between firms could halt that collaborative effort?
Participant 1: “I do. And that's probably the biggest concern from a defence point of view is that the companies will say I'm taking my big data away to spy on my own field and that would leave either one company wanting to get a monopoly on the system.”
It is out of the scope of this studies research aims to investigate the potential economic and political motivations for companies not to share their CS attack data, however this should be considered a topic for future research as to identify the cause for this lack of co-operation from private firms. The above evidence, however, does reveal a fourth challenge to the implementation of DS methods to CS practices:
· There is a lack of quality labelled datasets to train and validate CS ML methods on.
Time Sensitivity
Time sensitivity was the most observed theme in both policy documents and interview transcripts (N=48). This theme included the reaction times in AI vs humans to CS threats and the timescales in which these new technologies are growing. As identified in the literature review, current systems reliant on humans may be inadequate to detecting suspicious internal network traffic(Anwar, et al., 2017), but combining these methods with automated IDS may provide more timely security than traditional human reliant systems in the future(Amine Ferrag, Maglaras, Moschoyiannis, & Janicke, 2020). The Cybersecurity for power systems 114th congress policy document revealed that by the time an adversary penetrates your environment/network, they’re not the actor that you see(United States Congress, 2015), meaning when infiltrated it is difficult for a network to then spot a hacker from within. This confirms the need for timely response to cyber-attacks. AI is helping detect new assaults faster than humans and predicting potential future attack vectors but also helping combat against new AI powered threats(Dash, Farheen Ansari, Sharm, & Ali, 2022). This presents a fifth challenge:
· Companies need to embrace modern AI defence systems to keep up with emerging threats or be left behind with old systems with insufficient reaction times.
Conclusion
Evidenced through thematic analysis of 8 policy documents and 2 interviews with CS professionals, 5 sub-themes across the documents were revealed. Upon discussion of the sub-themes evidenced by existing literature, 5 challenges that related to the adoption of DS methods (AI, ML) in CS practices were discovered:
1. The questionable accuracy of ML methods within an IDS to detect an adversarial AI threat, considering the consequences of an anomaly.
2. The threat posed by advances of AI adapted code surpassing current defence mechanisms.
3. The balance between technological innovation and policies overseeing these technologies.
4. There is a lack of quality labelled datasets to train and validate CS ML methods on.
5. Companies need to embrace modern AI defence systems to keep up with emerging threats or risk being left behind with old systems with insufficient reaction times.
In line with research objective 4, from these 5 challenges the following principles can be suggested for policy makers to consider:
· Ensure full disclosure to the relevant governing bodies of any AI/ML methods used within CS practices including training data, accuracy results, model used, and methodology. Furthermore, make the training data used accessible to other companies within the same field upon approval.
· Make policies adaptable and proactive against the ever-changing nature of AI. But also reviewing these policies quarterly to keep up with innovation.
Conclusion
Limitations
With the chosen methodology 4 main themes were identified Critical Infrastructures, Cybersecurity Organisations, Cybersecurity Technique(s), and Data Science Methods. These themes did not contribute much to the research aim or objectives alone, but it was the subsequent sub-themes within the Data Science methods theme which contributed to the research aim. Upon consideration a code book may be more appropriate for providing more structure and consistency to the themes discovered, allowing the main themes to contribute more to the ideation of theories on challenges found(Oliveira, 2021). However as discussed before the inductive approach was chosen for its ability to allow for flexibility in themes discovered to allow for further insight on the themes discovered research(Braun & Clarke, 2006).
Another limitation to be considered was that the collection of policy documents used in the discussion may not be complete or representative of the overall landscape of the area the policy documents are representing(Cardno, 2018). These policy documents are part of a much larger web that inform procedures and guidelines for implementation that radiate from these original policy documents and impact how the policies are implemented at an organisational level(Cardno, 2018). However, it was beyond the timeframe and resources of this study for one to conduct such extensive research in not only expanding the policy document pool but to include procedures and guidelines formed from these.
It is acknowledged the precise applications of this study to power grids could be questioned. All policy documents related directly to the implementation of CS within national power grids, however not all documents mentioned machine learning methods (see figure 6). Furthermore, it can be identified in the participant transcripts that neither currently work for a national power grid, but both however do work in critical infrastructure (Rail and Maritime Transport).
Summary
The aim of this study was to investigate the challenges, benefits, and consequences of the application of DS methods (AI and ML) to CS practices of power grids, the study found 5 challenges facing the fusion of these two domains to combat new threats facing our critical infrastructures’ security. Furthermore, it has provided areas for further research to be conducted and provided valuable industry knowledge from professionals within the field.
The literature review conducted provided a comprehensive overview of the current and emerging field of CSDS, establishing how machine learning techniques are used in CS, the devastating impact of CS attacks and, acknowledged previous foundational studies and their limitations. Henceforth achieving the first research objective to identify key issues relating to CS of power grids and how DS can help with combatting these issues.
With an established critical realist philosophy and qualitative inductive research approach for the methodology, the research objectives 2 and 3 were both achieved. Thematic analysis of 8 policy documents and 2 professional interview transcripts conducted revealed 4 main themes of discussion, with the descriptive statistics of the sub-themes and subsequent discussion shining light on some benefits and 5 challenges facing the CS industry with the adoption of DS methods. All whilst ensuring a confidential and ethical approach was taken for the participants to ensure their anonymity upon their consent. Through discussion of these challenges evidenced by past literature and developed through the semi structured interviews research objective 4 was achieved by identifying these challenges for professionals to ensure proper implementation of CSDS.
Suggestions for Further Research
Fulfilling the research question and aim of this paper, the challenges discovered have raised further questions for policy makers, organisations, and CS professionals to consider when implementing AI and ML methods into their CS practices. This has paved the way for further areas of research needed:
· How to improve the accuracy of ML methods within IDS systems to detect an adversarial AI threat?
· Identifying the root cause for the lack of cooperation between companies to share CS attack data.
· A potential framework for companies to follow for when implementing AI defence systems to keep up with emerging threats safely and timely?
Bibliography
A. Adeoye-Olatunde, O., & Olenik, N. (2021). Research and scholarly methods: Semi-structured interviews. Journal of the American College of Clinical Pharmacy, 1358-1367.
Aalst, v. (2016). Process mining: data science in action. Heidelberg: Springer.
Adams, W. (2015). Handbook of Practical Program Evaluation. In J. Wholey, H. Hatry, & K. E. Newcomer, Handbook of Practical Program Evaluation (pp. 492-505). Wiley.
Ahmad, T., & Zhang, D. (2021). Using the internet of things in smart energy systems and networks. Sustainable Cities and Society, 1-22.
Amine Ferrag, M., Maglaras, L., Moschoyiannis, S., & Janicke, H. (2020). Deep Learning for Cyber Security Intrusion. Journal of Information Security and Applications, 1-20.
Amine Ferrag, M., Maglaras, L., Moschoyiannis, S., & Janicke, H. (2020). Deep learning for cyber security intrusion detection: Approaches, datasets, and comparative study. Journal of Information Security and Applications, 1-20.
Andresen, A. X., Kurtz, L., Hondula, D., Meerow, S., & Gall, M. (2023). Understanding the social impacts of power outages in North America: a systematic review. Enviromental Research Letters, 1-15.
Antak, C., Billig, M., Edwards, D., & Potter, J. (2002). Discourse analysis means doing analysis: a critique of six analytic shortcomings. DAOL Discourse Analysis Online , 1.
Anwar, S., Mohamad Zain, J., Fadli Zolkipli, M., Inayat, Z., Khan, S., Anthony, B., & Chang, V. (2017). From Intrusion Detection to an Intrusion Response System: Fundamentals, Requirements, and Future Directions. Algorithms, 1-24.
Armerding, T. (2023, March 24). Obama’s cybersecurity legacy: Good intentions, good efforts, limited results. Retrieved from csoonline: https://www.csoonline.com/article/3162844/obamas-cybersecurity-legacy-good-intentions-good-efforts-limited-results.html
Astley, W., & Van de Ven, A. (1983). Central perspectives and debates in organization theory. Administrative Science Quarterly, 245-273.
Barth, B. (2023, July 15). Researchers bypass Cylance’s AI-based AV solution by masking malware with video game code. Retrieved from SC Media: https://www.scmagazine.com/news/malware/researchers-bypass-cylances-ai-based-av-solution-by-masking-malware-with-video-game-code
Bechor, T., & Jung, B. (2019). Current state and modeling of research topics in cybersecurity and data science. SYSTEMICS, CYBERNETICS AND INFORMATICS, 129-156.
Bedi, G., Kumar Venayagamoorthy, G., Singh, R., Brooks, R., & Wang, K.-C. (2018). Review of Internet of Things (IoT) in Electric Power and Energy Systems. IEEE Internet of Things Journal (Volume: 5, Issue: 2, April 2018), 847-870.
Beninger, K. (2017). Social Media Users’ Views on the Ethics of Social Media Research. The SAGE Handbook of Social Media Research Methods, 57-73.
Blackberry. (2023, March 23). Cylance AI. Retrieved from Blackberry: https://www.blackberry.com/us/en/products/cylance-endpoint-security/cylance-ai
Bowen, G. A. (2009). Document Analysis as a Qualitative. Qualitative Research Journal, 27-38.
Boyd, D. (2010). Privacy and publicity in the context of big data. In Keynote Talk of The 19th Int’l Conf. on World Wide Web (Vol. 650).
Brahmi, I., Brahmi, H., & Ben Yahia, S. (2015). A Multi-agents Intrusion Detection System Using. 5th International Conference on Computer Science and Its, 381-393.
Brand-Correa , L., & Steinberger, J. (2017). A Framework for Decoupling Human Need Satisfaction From Energy Use. Ecological Economics, 43-52.
Braun, V., & Clarke, V. (2006). Using thematic analysis in psychology. Qualitative Research in Psychology, 77-101.
Braun, V., & Clarke, V. (2012). Thematic Analysis. APA handbook of research methods in psychology, Vol. 2. Research designs: Quantitative, qualitative, neuropsychological, and biological, 57-71.
Braun, V., & Clarke, V. (2012). THEMATIC ANALYSIS. APA Handbook of Research Methods in Psychology: Vol. 2. Research Designs,, 57-71.
Brian, C., William, D., Mark, J., & Baird, W. (2004). The Economic Impact of Cyber-Attacks. CRS Report for Congress: The Library of Congress. Retrieved July 15, 2023, from https://archive.nyu.edu/bitstream/2451/14999/2/Infosec_ISR_Congress.pdf.
Buchanan, D., & Bryman, A. (2009). The SAGE Handbook of Organizational Research Methods. London: Sage.
Buczak, A., & Guven, E. (2016). A Survey of Data Mining and Machine Learning Methods for Cyber Security Intrusion Detection. IEEE COMMUNICATIONS SURVEYS & TUTORIALS, 1153-1177.
Cachia, M., & Millward, L. (2011). The telephone medium and semi‐structured interviews: a complementary fit. Qualitative Research in Organizations and Management, 265-277.
Cardno, C. (2018). Policy Document Analysis: A Practical Educational Leadership Tool and a Qualitative Research Method. Educational Administration: Theory and Practice, 623-640.
Cashell, B., D. Jackson, W., Jickling, M., & Webel, B. (2004). The Economic Impact of Cyber-Attacks. Washington, D.C.: Congressional Research Service, The Library of Congress.
Castillo, A. (2014). Risk analysis and management in power outage and restoration: A literature survey. Electric Power Systems Research, 9-15.
Chen, T. F., Gisev, N., & Bell, J. (2013). Interrater agreement and interrater reliability: Key concepts, approaches, and applications. Research in Social and Administrative Pharmacy,, 330-338.
Chio, C., & Freeman, D. (2018). Machine Learning and Security: Protecting Systems with Data and Algorithms. London: O'Reilly.
Chio, C., & Freeman, D. (2018). Machine Learning and Security: Protecting Systems with Data and Algorithms. London: O'Reilly (WILEY UK).
Congressional Research Service. (2017). Cybersecurity for Energy Delivery Systems: DOE Programs. Washington D.C.: Congressional Research Service.
Craigen, D., Diakun-Thibault, N., & Purse, R. (2014). Defining Cybersecurity. Technology Innovation Management Review, 13-21.
CSIS. (2023, March 07). Significant Cyber Incidents. Retrieved from Center for Strategic and International Studies: https://www.csis.org/programs/strategic-technologies-program/significant-cyber-incidents
Darktrace. (2023, August 06). heal. Retrieved from Darktrace: https://darktrace.com/products/heal
Dash, B., Farheen Ansari, M., Sharm, P., & Ali, A. (2022). Threats and Opportunities with AI-Based Cyber Security Intrusion Detection: A Review. International Journal of Software Engineering & Applications, 13-22.
Dhakal, K. (2022). NVivo. Journal of the Medical Library Association : JMLA, 110, 270–272.
EECSP. (2017). Recommendations for the European Commission on a European Strategic Framework and Potential Future Legislative Acts for the Energy Sector. EECSP Expert Group.
European Commission. (2013). Cybersecurity Strategy of the European Union: An Open, Safe and Secure Cyberspace, Report. Joint Communication to the European Parliament, the Council, the European Economic and Social Committee and the Committee of the Regions.
European Union. (2023, March 11). Council Directive 2008/114/EC of 8 December 2008 on the Identification and Designation of European Critical Infrastructures. Retrieved from EUR-Lex: https://eur-lex.europa.eu/legal-content/en/ALL/?uri=CELEX:32008L0114
European Union. (2023, July 15). timeline-cybersecurity. Retrieved from Consilium: https://www.consilium.europa.eu/en/policies/cybersecurity/timeline-cybersecurity/
Fang, X., Misra, S., Xue, G., & Yang, D. (2011). Smart Grid — The New and Improved Power Grid: A Survey. IEEE Communications Surveys & Tutorials, 944-980.
Fielden, A., Silence, E., & Little, L. (2011). Children's understandings’ of obesity, a thematic analysis. Int J Qual Stud Health Well-being.
G. Carbonell, J., S. Michalski, R., & M. M, T. (1983). Machine Learning. Salt Lake City: Elsevier Inc.
Gartner. (2023, August 02). Gartner Predicts Nearly Half of Cybersecurity Leaders Will Change Jobs by 2025. Retrieved from Gartner: https://www.gartner.com/en/newsroom/press-releases/2023-02-22-gartner-predicts-nearly-half-of-cybersecurity-leaders-will-change-jobs-by-2025
Goel, S., Guleria, K., & Narayan Panda, S. (2022). Anomaly based Intrusion Detection Model using Supervised Machine Learning Techniques. 10th International Conference on Reliability, Infocom Technologies and Optimization (Trends and Future Directions), 1-5.
Gubbi, J., Buyya, R., Marusic, S., & Palaniswami, M. (2013). Internet of Things (IoT): A vision, architectural elements, and future directions. Future Generation Computer Systems, 1645-1660.
Habibzadeh, H., H. Nussbaum, B., Anjomshoa, F., Kantarci, B., & Soyata, T. (2019). A survey on cybersecurity, data privacy, and policy issues in cyber-physical system deployments in smart cities. Sustainable Cities and Society, 1-20.
Han, D., Zhang, J., Zhang, Y., & Gu, W. (2010). Convergence of Sensor Networks/Internet of Things and Power Grid Information Network at Aggregation Layer. International Conference on Power System Technology, 1-6.
Hathaway, O., Crootof, R., Levitz, P., Nix, H., Nowlan, A., Perdue, W., & Spiegel, J. (2012). The Law of Cyber-Attack. California Law Review. 100(4), 817-885.
Hove, S., & Anda, B. (2005). Experiences from conducting semi-structured interviews in empirical software engineering research. 11th IEEE International Software Metrics Symposium (METRICS'05), 10-23.
Humayun, M., Jhanjhi, N., Talib, M., Shah, M., & Suseendran, G. (2021). Cybersecurity for Data Science: Issues, Opportunities, and Challenges. Intelligent Computing and Innovation on Data Science, 435-443.
Jensen , K. (2002). A Handbook of Media and Communication Research – Qualitative and Quantitative Methodolo-gies. In K. Jensen, The Qualitative Research Process (pp. 235-254). London and New York: Routledge.
Kelleher, J. D., & Tierney, B. (2018). Data Science. Cambridge, Massachusetts: MIT Press.
Kirkegaard, E. O., & Bjerrekær, J. D. (2016). The OKCupid dataset: A very large public dataset of dating site users. Open Differential Psychology, 1-19.
Kitchin, R. (2021). The Data Revolution A Critical Analysis of Big Data, Open Data and Data Infrastructures. Ireland: Sage.
Komninos, N., Philippou, E., & Pitsillides, A. (2014). Survey in smart grid and smart home security: Issues challenges and countermeasures. IEEE Commun. Surveys Tuts, 1933-1954.
Krause, T., Ernst, R., Klaer, B., Hacker, I., & Henze, M. (2021). Cybersecurity in Power Grids: Challenges and Opportunities. Sensors, 1-19.
Kumar Venkatachary, S., Prasad, J., & Samikannu, R. (2017). Economic Impacts of Cyber Security in Energy Sector: A Review. International Journal of Energy Economics and Policy, 250-262.
L. Bayuk, J., Healey, J., Rohmeyer, P., H. Sachs, M., Schmidt, J., & Weiss, J. (2012). Cyber Security Policy Guidebook. New York: John Wiley & Sons.
Lewis, J. A. (2006). Cybersecurity and Critical Infrastructure Protection. Washington, DC:: Center for Strategic and International Studies. Retrieved from Washington, DC: Center for Strategic and International Studies.: https://www.csis.org/analysis/cybersecurity-and-critical-infrastructure-protection
Li, S., Da Xu, L., & Zhao, S. (2015). The internet of things: a survey. Inform Syst Front, 243-259.
Lu, R., Zhu, H., Liu, X., Liu, J., & Shao, J. (2014). Toward efficient and privacy-preserving computing in big data era. IEEE, 46-50.
Lv, H., & Tang , H. (2011). Machine Learning Methods And Their Application Research . International Symposium on Intelligence Information Processing and Trusted Computing, 1-3.
Madakam, S., Ramaswamy, R., & Tripathi, S. (2015). Internet of Things (IoT): A Literature Review. Journal of Computer and Communications, 1-10.
Marsden, P. (1996). Procedures in the nuclear industry. Human factors in Nuclear Safety, 99-116.
Mazhelis, O., Luoma, E., & Warma, H. (2012). Defining an Internet-of-Things Ecosystem. Internet of Things, Smart Spaces, and Next Generation Networking (pp. 1-14). Berlin Heidelberg: Springer.
Meserve, J. (2023, March 23). Mouse click could plunge city into darkness, experts say. Retrieved from CNN: http://edition.cnn.com/2007/US/09/27/power.at.risk/index.html
Miller, K., & Tsang, E. (2010). TESTING MANAGEMENT THEORIES: CRITICAL REALIST PHILOSOPHY AND RESEARCH METHODS. Strategic Management Journal, 139-158.
Mills, M. P. (2016). EXPOSED: How Americas Electric Grids are Becoming Greener, Smarter - and More Vulnerable. Washington D.C: Manhattan Institute.
Milmo, D. (2023, March 25). NHS ransomware attack: what happened and how bad is it? Retrieved from theguardian: https://www.theguardian.com/technology/2022/aug/11/nhs-ransomware-attack-what-happened-and-how-bad-is-it
Murkowski, M. (2018). SECURING ENERGY INFRASTRUCTURE ACT. Washington D.C.: United States Congress.
Nardelli, P., Rubido, N., Wang, C., Baptista, M., Pomalaza-Raez, C., Cardieri, P., & Latva-aho, M. (2014). Models for the modern power grid. The European Physical Journal Special Topics, 2423-2437.
NCSC. (2023, August 04). Principles for the security of machine learning. Retrieved from National Cyber Security Centre: https://www.ncsc.gov.uk/collection/machine-learning/development-prerequisites-and-wider-considerations/design-for-security
Nicholas, J., & Goodwin, C. (2015). A framework for cybersecurity information sharing and risk reduction. Microsoft.
Oliveira, G. (2021). Developing a codebook for qualitative data analysis: insights from a study on learning transfer between university and the workplace. International Journal of Research & Method in Education, 300-312.
Ou, Q., Zhen, Y., Li, X., Zhang, Y., & Zeng, L. (2012). Application of Internet of Things in Smart Grid Power Transmission. International Conference on Mobile, Ubiquitous, and Intelligent Computing (pp. 96-100). Beijing, China: IEEE.
P. Sanghvi, A. (1991). Power shortages in developing countries: Impacts and policy implications. Energy Policy, 425-440.
Pala, A., & Zhuang, J. (2019). Information Sharing in Cybersecurity: A Review. Decision Analysis, 172-196.
Palensky, P., & Kupzog, F. (2013). Smart Grids. The Annual Review of Environment and Resources, 201-226.
Participant 1. (2023, July 20). Interview for Dissertation: The Challenges of Implementing Data Science Methods to the Cybersecurity. (C. Brazier, Interviewer)
Participant 2. (2023, July 11). Interview for Dissertation: The Challenges of Implementing Data Science Methods to the Cybersecurity. (C. Brazier, Interviewer)
Patanakul, P., & K. Pinto, J. (2014). Examining the roles of government policy on innovation. The Journal of High Technology Management Research, 97-107.
Peng, C., Xu, M., Xu, S., & Hu, T. (2018). Modeling multivariate cybersecurity risks. Journal Of Applied Statistics, 2718-2740.
Perrault, R., Brynjolfsson, E., Clark, J., Etchemendy, J., Grosz, B., Lyons, T., . . . Mishra, S. (2019). Artificial Intelligence Index. Stanford, California: Stanford University.
Popper, K. (1959). The Logic Of Scientific Discovery. New york: Basic Books.
Punch, K. (2004). Developing Effective Research Proposals. London: SAGE.
Reilly, P. (2014). The ‘Battle of Stokes Croft’ on YouTube: The. SAGE Research Methods Cases Development of an Ethical Stance for the Study of Online Comments, 1-13.
Sarkar, S., Teo Meng, Y., & Chang, E.-C. (2022). A cybersecurity assessment framework for virtual operational technology in power system automation. Simulation Modelling Practice and Theory, 1-17.
Sarker, H. I., Kayes, M. A., Badsha, S., Alqahtani, H., Watters, P., & Ng, A. (2020). Cybersecurity data science: an overview from machine learning perspective. Journal of Big Data, 1-29.
Sayer, A. (2000). Realism and Social Science. London: Sage.
Scott, J. (1990). Documentary Sources in Social Research. Cambridge: Polity.
Singh, A., Venter, H., & Adeyemi Ikuesan, R. (2022). Ransomware Detection using Process Memory. Proceedings of the 17th International Conference on Information Warfare and Security, 413-423.
Soifer, D., & Goure, D. (2014). Keeping the lights on: How Electricity Policy Must Keep Pace with Technology. Arlington: Lexington Institute.
Soni, T., Kaur, R., Gupta, D., Sharma, A., & Gupta, G. (2023). The Cybersecurity Ecosystem: Challenges, Risk and Emerging Technologies. IEEE, 1-60.
Sturges, J., & Hanrahan, K. (2004). Comparing telehpone and face-to-face qualitative interviewing: a research note. Qualitative Research, 107-118.
Suhr, J., Cutrona, C., Krebs, K., & Jensen, S. (2004). Couple Observational Coding Systems. Routledge.
Tang, M., Alazab, M., IEEE, & Luo, Y. (2019). Big Data for Cybersecurity: Vulnerability Disclosure Trends and Dependencies. IEEE TRANSACTIONS ON BIG DATA, 635-647.
Tessian. (2023, August 06). platform. Retrieved from Tessian: https://www.tessian.com/platform/
Trevisan, D., & Reilly, D. (2022, June 10). UKIP: The web’s darling? Retrieved from Election Analysis: https://www.electionanalysis.uk/uk-election-analysis-2015/section-6-social-media/ukip-the-webs-darling/
Turing, A. (1942). The Applications of Probability to Cryptography. National Archives.
United States Congress. (2015). Cybersecuirty for Power Systems. Congress of the United States House of Representitives Committee on Science, Space and Technology (pp. 6-102). Washington DC: Congress of the United States.
University of Wisconsin Data Science Team. (2022, December 29). A Modern History of Data Science. Retrieved from University of Wisconsin Data Science : https://datasciencedegree.wisconsin.edu/blog/history-of-data-science/
Vaismoradi, M., Turunen, H., & Bondas, T. (2013). Content analysis and thematic analysis: Implications for conducting a qualitative descriptive study. Nursing and Health Sciences, 398-405.
van Dijck, J. (2014). Datafication, dataism and dataveillance: Big Data between scientific paradigm. Surveillance & Society, 197-208.
Venkatachary, S., Prasad, J., & Samikannu, R. (2017). Economic Impacts of Cyber Security in Energy Sector: A Review. International Journal of Energy Economics and Policy, 250-262.
Walker, D., & Myrick, F. (2006). Grounded Theory: An Exploration of Process and Procedure. QUALITATIVE HEALTH RESEARCH, Vol. 16 No. 4,, 547-559.
Whitlock, P. (2023, August 04). ARTIFICIAL INTELLIGENCE: THE NEXT EVOLUTION IN CYBER THREAT DETECTION? Retrieved from isc2: https://blog.isc2.org/isc2_blog/2023/06/artificial-intelligence-the-next-evolution-in-cyber-threat-detection.html
Xin, Y., Kong, L., Liu, Z., Chen, Y., Li, Y., Zhu, H., . . . Wang, C. (2018). Machine Learning and Deep Learning Methods for Cybersecurity. IEEE Access, 35365-35381.
Yoonsang, K., Huang, J., & Emery, S. (2016). Garbage in, Garbage Out: Data Collection, Quality Assessment and Reporting Standards for Social Media Data Use in Health Research, Infodemiology and Digital Disease Detection. Journal of Medical Internet Research.
Zarpelao, B., Miani, R., Kawakani, C., & de Alvarenga, S. (2017). A survey of intrusion detection in internet of things. Journal of Network and Computer Applications, 25-37.
Zhang, J., Rimba , P., Gao , S., Zhang, L., Xiang, Y., & Sun, N. (2018). Data-driven cybersecurity incident prediction: a survey. IEEE Commun Surv Tutor., 1744-1772.
Zimmer, M. (2010). ‘‘But the data is already public’’: on the ethics of research in Facebook. Ethics Inf Technol, 313-325.
Comments ()